Generative Artificial Intelligence (AI) represents a frontier in the evolution of machine learning and artificial intelligence technologies. Unlike traditional AI systems programmed to perform specific tasks, generative AI goes a step further; it creates. This ability to generate new content, from realistic images to complex written text and innovative music compositions, is not just transforming industries but also reshaping our understanding of creativity.
The allure of generative AI lies in its vast potential across various sectors—be it enhancing creativity in the arts, speeding up drug discovery in pharmaceuticals, or providing personalized experiences in media and entertainment. As we delve deeper into this exciting technology, we’ll explore how it works, its applications, and the profound impact it promises on both society and industry.
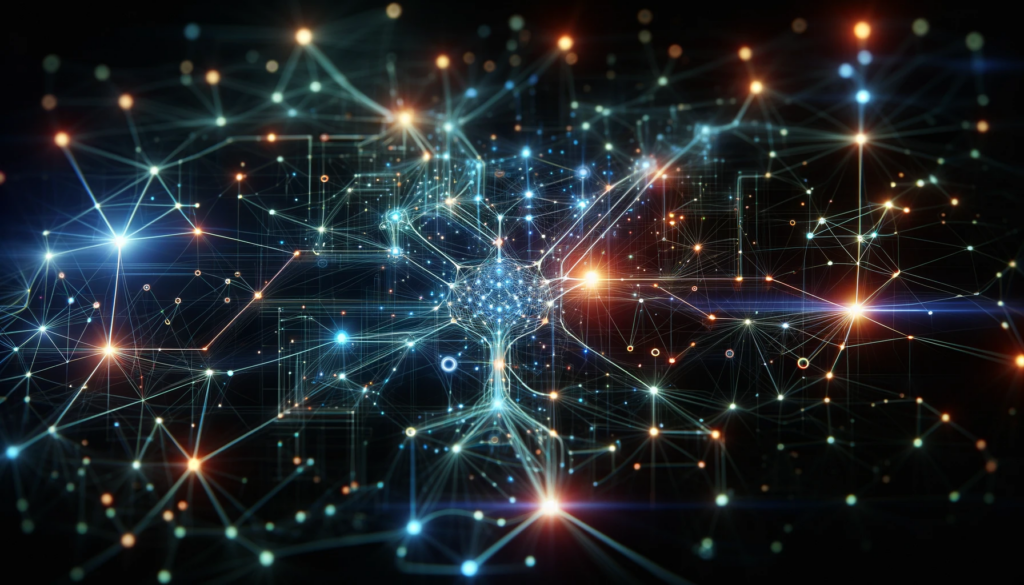
How Generative AI Works
Generative AI uses models that learn to create content similar to their training data. At the core of generative AI are two popular model types: Generative Adversarial Networks (GANs) and Variational Autoencoders (VAEs).
Generative Adversarial Networks (GANs) consist of two parts: the generator and the discriminator. The generator creates images, texts, or other data forms from random noise, while the discriminator evaluates them against the real data, learning to distinguish between the generated and the actual data. This competitive process improves the quality of the generated outputs significantly over time, making the fakes indistinguishable from real data.
Variational Autoencoders (VAEs) encode input data into a smaller, dense representation and use it to generate new data similar to the original after decoding. VAEs excel at creating new images or reconstructing damaged ones.
These models learn from vast datasets, with output quality depending on data volume and variety. Their complexity allows adaptation to many applications, from creating new fonts to simulating human behavior in digital environments.
Applications of Generative AI
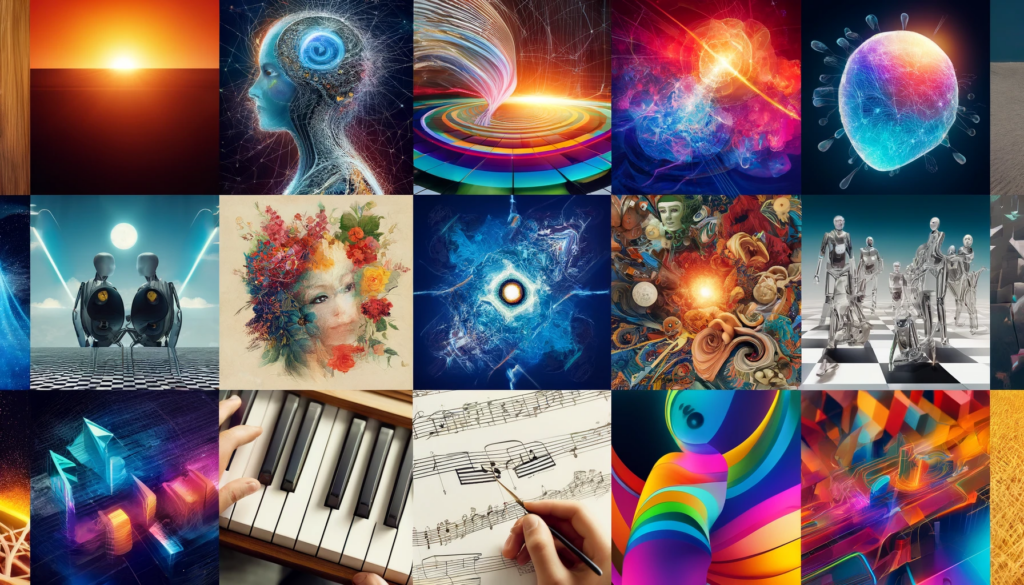
Generative AI’s ability to create and innovate makes it incredibly versatile. Here are a few applications where it is making a mark:
- Art and Design: Artists are using tools like DeepDream and DALL-E to create intricate artwork that blurs the line between human and machine creativity.
- Music: AI systems like AIVA (Artificial Intelligence Virtual Artist) compose original music scores that are used in movies, games, and even by symphony orchestras.
- Healthcare: In drug discovery, generative models are used to predict molecular structures that could lead to new pharmaceuticals.
- Content Creation: From writing assistive tools that help generate articles, stories, and poetry to video games where AI creates dynamic narratives.
Benefits of Generative AI
The advantages of using generative AI are manifold:
- Efficiency and Speed: AI can generate data and simulations much faster than human counterparts, speeding up research and development across various fields.
- Scalability: Once a model is trained, it can generate countless outputs without additional costs, making it highly scalable.
- Customization: Generative AI can produce tailored content that fits specific customer preferences, enhancing personalization in services and products.
Challenges and Ethical Considerations
However, generative AI is not without its challenges:
- Ethical Concerns: There is a growing debate about the ethical implications of AI in the creation process, including issues of copyright when AI replicates styles or content.
- Bias and Fairness: AI models can inherit and amplify biases present in their training data, leading to unfair outcomes.
- Data Privacy: Using sensitive data to train AI models raises concerns about privacy and security, especially when the data can be reverse-engineered from AI-generated content.
The Future of Generative AI
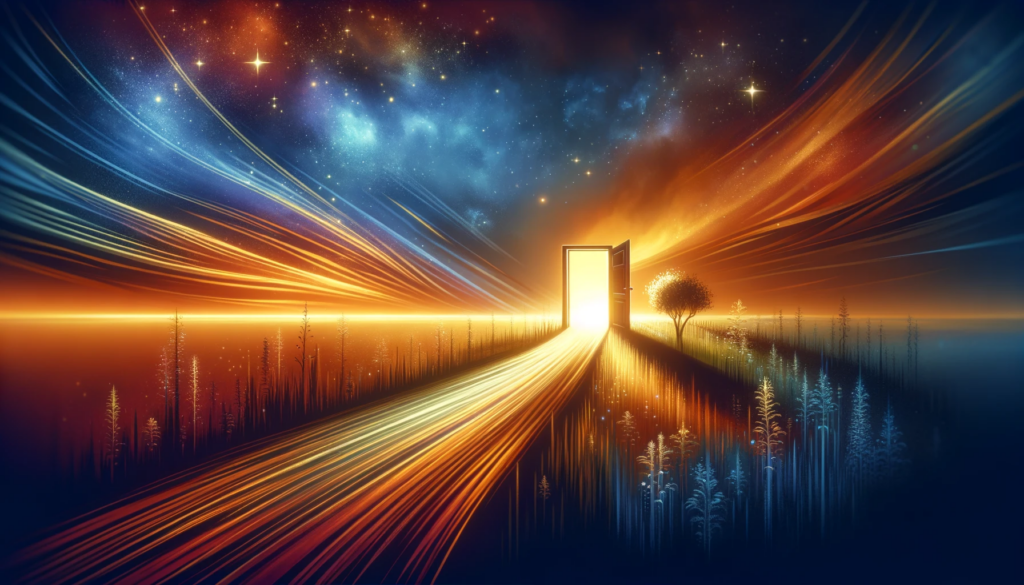
As AI evolves, it will integrate more into daily life with personalized assistants, smarter content curation, and AI in governance. Strong ethical frameworks and regulations are essential.
Conclusion
Generative AI is rapidly advancing and highly exciting. By understanding its mechanisms, applications, and impacts, we can harness its capabilities and address its challenges. As we enter this AI-driven era, both the possibilities and responsibilities are immense.